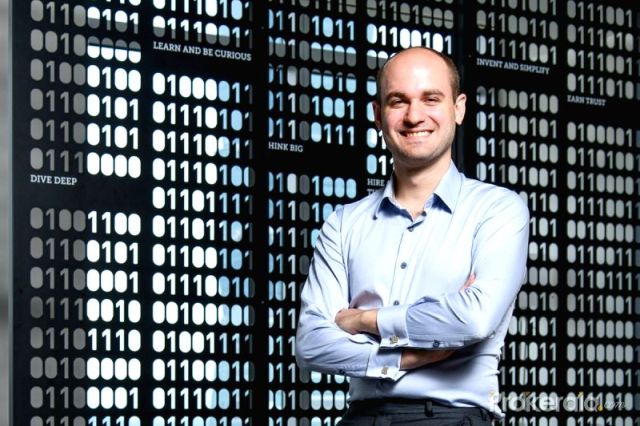
Training machines sans bias will only augment humans: AWS executive
By Nishant Arora,
New Delhi : At a time when the debate over machines replacing humans rages, a top Amazon Web Services (AWS) executive is convinced that machines are not here to take decisions on their own, and certain human emotions — empathy, for instance — cannot be automated.
Last year, Facebook Artificial Intelligence Researchers (FAIR) had to shut down one of its Artificial Intelligence (AI) systems after chatbots started speaking in their own language, defying the codes provided.
There have been other instances too where anomalies in the AI models were noticed.
However, according to Olivier Klein, Head of Emerging Technologies, Asia-Pacific, at AWS, which is retail giant Amazon’s Cloud arm, a Machine Learning (ML) model will always operate the way you’ve trained it.
“If you train a model with a bias, you would end up with a biased model. You continuously need to train and re-train your ML model and the most important thing is that you need some form of feedback from the end-consumers,” Klein told IANS in an interview.
“I think there are certain elements of human emotions like empathy that cannot be automated. There will be scenarios where it makes sense to automate and give customers better experiences. ML is absolutely not about replacing humans but enhancing the experiences,” he explained.
The success depends on the data points, or observations, that you put into the deep learning or neural networks.
“If your data points are very small and minimal, you probably end up with a model that is not really doing what you want it to do. So it always goes back to what’s the data that you’re collecting and what are you training ML models on,” explained Klein.
At AWS, he and the team is busy adding unbiased data inputs to ML models, building services around those for enhanced consumer experience.
“We keep training and retraining the ML models and optimising those. Take, for example, our Amazon Rekognition service. It has a variety of different capabilities like object detection, object recognition, sentiment detection, etc. One of it is also facial recognition,” said the AWS executive.
“Recently, we updated our capabilities to increase the accuracy that we have within the facial recognition space to have a 40 per cent higher accuracy than we previously had,” Klein noted.
He stressed that the outcome of AI/ML models are always based on the data and the training with those.
“Training the ML models is not a one-time effort,” he said, adding human supervision is required to annotate the customers’ feedback and say, “we should retrain these kind of data points because they are not performing the way they should”.
To create better AI/ML models, one has to look at different data sets, write out the bias in the data set and have an unbiased model in the end.
“Your first model really isn’t perfect but then, from there on, you keep increasing the accuracy and the performance, depending on what the use case is,” Klein explained.
When it comes to humans, they are good in dealing with situations that have ambiguous kind of data points.
“Humans are really good at learning quickly with very little information. ML models are the opposite. They require a lot of data inputs to be able to be trained.
“I would argue that you show someone a bicycle a few times and you show them how to ride a bicycle after few times the human being is able to ride that bicycle pretty easily. To just train a robot to ride a bicycle takes millions of hours of training,” explained Klein.
In the last one year, AWS has released over 200 ML services and features.
When it comes to Amazon Alexa now talking to humans, he said lot of their customers are using the platform to do voice profiling for a variety of reasons.
“For example, in the financial services industry, we have customers that are looking into voice profiling as an additional factor at their call centres. So if they want to verify if it’s you, they can add voice profiling as an additional factor to further reduce fraudulent or impersonation calls,” he explained.
In a nutshell, Klein said, ML would only complement and enhance the human work once bias is out from our minds.
(Nishant Arora can be contacted at nishant.a@ians.in)
—IANS